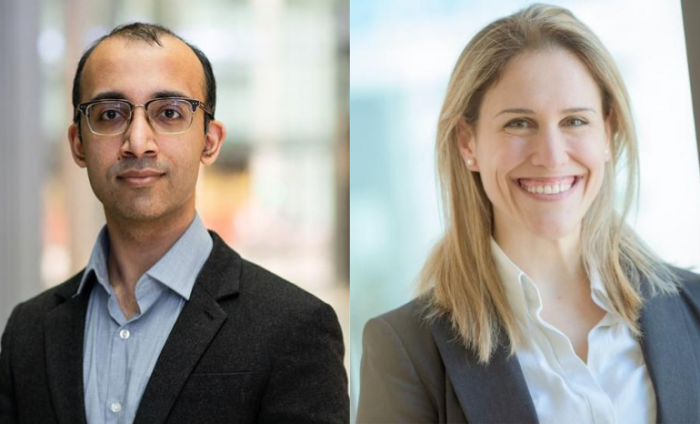
A recent study from UCL School of Management PhD candidate and Adjunct Lecturer Shivaang Sharma and Assistant Professor Angela Aristidou maps the contours of AI responsibility rifts and unveils the frictions and expectations, values, and perspectives about the ethicality of AI tools amongst the stakeholders using them.
The study will be published in the forthcoming 2025 MIS Quarterly Executive and, while much is said about the objective risks of AI - such as hallucinations, malicious misuse, or cybersecurity threats - the research in this study addresses a deeper dissonance: AI Responsibility Rifts (AIRR).
Defining AI Responsibility Rifts: Beyond the Risk Lens
The dominant approach to managing AI has revolved around mitigating risks—technical or operational threats that can be preemptively identified and addressed. These include biases in training data, unintended algorithmic outputs, or safety thresholds for AI behavior. While the AI risk lens is invaluable, it is inherently technocentric, focusing primarily on issues that can be resolved through governance, explainability mechanisms, or adversarial training techniques.
In contrast, the AIRR lens uncovers rifts—persistent disagreements among stakeholders about the ethicality and societal impact of an AI tool’s design, implementation, and effects. These dissonances occur because stakeholders—such as developers, users, regulators, and affected communities—experience AI differently, shaped by their unique interactions and social contexts. For example, while developers might prioritize system transparency and accuracy, grassroots users may question whether a tool inadvertently deepens power asymmetries. These rifts are not merely technical disputes but moral quandaries, demanding nuanced solutions.
The SHARE framework, outlined in this study, identifies five interconnected dimensions of AI responsibility that are particularly prone to such rifts: Safety, Humanity, Accountability, Reliability, and Equity. Unlike risks, which are often visible and measurable, rifts emerge in the shadows of moral pluralism and sociotechnical divides, shaping whether AI tools are integrated, trusted, or discarded.
Unveiling the SHARE Dimensions
Safety: Contested Groundlines
Rifts over safety reflect disagreements on what constitutes “safe” AI use—debates fueled by cultural, technical, and operational divides. For instance, while technologists may emphasize encryption and fail-safes, frontline users might perceive safety through the lens of harm minimization to vulnerable communities.
Humanity: Risks of Dehumanisation
The ethical impact of AI on human dignity, identity, and relationships fuels rifts about its effect on humanity. Stakeholders deliberate whether a tool erodes empathy or reduces people to data points, especially in emotionally charged humanitarian contexts.
Accountability: Blurred Boundaries
Rifts regarding accountability revolve around uncertainty over who holds ultimate liability for AI-driven decisions. Stakeholders often face difficulty demarcating responsibility in systems where human and AI roles intertwine.
Reliability: A Trust Tightrope
Questions of AI reliability go beyond technical robustness to encompass user trust in its adaptability and appropriateness to diverse, dynamic scenarios. Differing views on whether AI outputs are genuinely context-sensitive create persistent dissonances.
Equity: Inclusive for Whom?
Equity rifts arise when AI tools disproportionately benefit or harm different groups. Concerns around inclusion in design and the representativeness of training data highlight this persistent ethical challenge.
Closing AI Responsibility Rifts: Practical Solutions for SHARE Dimensions
Safety: Building Guardrails for Consensus
- Partial Solution: Implement immediate safeguards, such as anomaly detection and transparent governance audits, to address concerns over misuse or unintended consequences.
- Holistic Approach: Engage local stakeholders in defining context-specific thresholds for safety that align with humanitarian principles. For example, human rights officers could co-develop protocols to protect identity data.
- Caveat: Developers must anticipate potential trade-offs between the need for data transparency and the risk of data misuse in high-stakes humanitarian contexts.
Humanity: Restoring Meaning and Empathy
- Partial Solution: Foster user-centric tools with customizable features that empower users rather than dictate outcomes. Introducing meaningful human-in-the-loop mechanisms reassures stakeholders of their decision-making autonomy.
- Holistic Approach: Organize participatory workshops where humanitarian aid workers and affected communities co-define what “humanity” means in AI applications, ensuring that these tools reflect shared values.
- Caveat: AI developers should remain wary of politicizing the concept of “humanity” across regions, as cultural interpretations could further alienate certain groups.
Accountability: Clarifying Ownership of Consequences
- Partial Solution: Develop transparent interaction logs that delineate actions performed by humans versus machine agents, enabling traceable accountability.
- Holistic Approach: Establish standing committees that bring together regulators, developers, and users to co-create shared accountability frameworks tailored to specific contexts, such as AI use in conflict zones.
- Caveat: As AI applications become more pervasive, ensuring accountability frameworks remain adaptable to new innovations is crucial.
Reliability: Reassuring Trust Through Context-Aware Design
- Partial Solution: Provide context-specific reliability demonstrations, showing how an AI tool adapts to local cultural and operational nuances.
- Holistic Approach: Develop user feedback loops where models learn from real-time corrections, fostering a system that evolves with user confidence and situational demands.
- Caveat: Balancing the transparency of AI decision-making with the complexity of underlying algorithms poses a persistent challenge for developers.
Equity: Embedding Inclusion from Design to Deployment
- Partial Solution: Integrate bias-detection modules during the development phase to highlight disparities in AI outputs.
- Holistic Approach: Establish long-term participatory processes with marginalized communities to ensure their values and needs are encoded into AI systems. This could include co-developing datasets or algorithms.
- Caveat: Equity initiatives must balance local specificity with scalable solutions, avoiding trade-offs that sacrifice inclusivity for efficiency.
Acknowledgements
This research was made possible through ongoing collaborations with United Nations Office for the Coordination of Humanitarian Affairs (UN OCHA), UN ReliefWeb, Data Friendly Space (DFS), iMMAP Inc and the Humanitarian AI community. We believe such research-practitioner collaborations are integral to addressing complex societal challenges at a time of escalating global crises.